郭晶晶
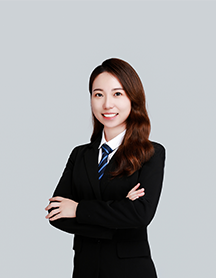 |
基本信息
姓名:郭晶晶
籍贯:湖南宁乡
学位:博士
职称:助理教授
专业方向:工程管理、智能建造
通讯地址:湖南省长沙市岳麓区麓山南路 湖南大学土木工程学院,410082
邮箱: guojingjing@hnu.edu.cn
|
教育背景
2014.9-2018.7,重庆大学,工程造价专业,获学士学位
2018.8-2022.3,新加坡国立大学,建筑环境专业(工程管理方向),获得博士学位。导师:王骞
工作履历
2022.6-至今,湖南大学土木工程学院,助理教授
2022.1-2022.6,新加坡国立大学建筑环境系,博士后
2018.8-2022.1,新加坡国立大学建筑环境系,研究助理
研究领域
- 智能建造与运维
- 建筑质量检测及健康监测
- 计算机视觉与土建工程的交叉应用
- 建造机器人
科研项目
[1]国家自然科学基金-青年科学基金项目(52308312,2024.1-2026.12),基于多源信息融合机制的桥梁病害智能识别与定位方法
[2]湖南省自然科学基金-企业联合基金(2023JJ70002,2023.1-2025.12),基于虚实映射的构件级别建筑施工进度自动感知方法研究
[3]新加坡国家教育部MOE Start-up Grant(2018.01-2020.12), Geometry quality inspection of PPVC/PBC using point cloud data and BIM,项目骨干
[4]新加坡国家教育部MOE Tier 1 Grant (2020.03-2022.06), Artificial intelligence assisted scan-to-BIM for existing buildings in A&A projects,项目骨干
学术兼职
《Advance Engineering Informatics》、《Journal of Building Engineering》、《Measurement》、《Building and Environment》等国际期刊审稿专家
代表性论文
[1]Guo, J., Wang, Q., Su, S., & Li, Y. (2023). Informativeness‐guided active learning for deep learning–based façade defects detection. Computer‐Aided Civil and Infrastructure Engineering.
[2]Guo, J., Wang, Q., Li, Y., & Liu, P. (2020). Façade defects classification from imbalanced dataset using meta learning‐based convolutional neural network. Computer‐Aided Civil and Infrastructure Engineering, 35(12), 1403-1418. https://doi.org/10.1111/mice.12578 (JCR一区, 中科院一区Top(升级版),中科院一区Top(基础版),IF = 10.066)
[3]Guo, J., Wang, Q., & Li, Y. (2021) Semi‐supervised learning based on convolutional neural network and uncertainty filter for façade defects classification. Computer‐Aided Civil and Infrastructure Engineering. 36(3), 302-317. https://doi.org/10.1111/mice.12632 (JCR一区, 中科院一区Top(升级版),中科院一区Top(基础版),IF = 10.066)
[4]Guo, J., Wang, Q., & Park, J. H. (2020). Geometric quality inspection of prefabricated MEP modules with 3D laser scanning. Automation in Construction, 111, 103053. https://doi.org/10.1016/j.autcon.2019.103053 (JCR一区, 中科院一区Top(升级版),中科院二区Top(基础版),IF = 10.517)
[5]Guo, J., Yuan, L., & Wang, Q. (2020). Time and cost analysis of geometric quality assessment of structural columns based on 3D terrestrial laser scanning. Automation in Construction, 110, 103014. https://doi.org/10.1016/j.autcon.2019.103014 (JCR一区, 中科院一区Top(升级版),中科院二区Top(基础版),IF = 10.517)
[6]Guo, J., Wang, Q., & Li, Y. (2021). Evaluation-oriented façade defects detection using rule-based deep learning method. Automation in Construction, 131, 103910. https://doi.org/10.1016/j.autcon.2021.103910 (JCR一区, 中科院一区Top(升级版),中科院二区Top(基础版),IF = 10.517)
[7]Guo, J. & Wang, Q. (2022). Human-related uncertainty analysis for automation-enabled façade visual inspection: a Delphi study. Journal of Management in Engineering (ASCE), 38(2), 04021088. https://doi.org/10.1061/(ASCE)ME.1943-5479.0001000 (JCR一区, 中科院二区(升级版),中科院三区(基础版),IF = 6.415)
[8]Wang, Q., Guo, J., & Kim, M. K. (2019). An application oriented scan-to-BIM framework. Remote sensing, 11(3), 365. https://doi.org/10.3390/rs11030365 (JCR一区, 中科院二区Top(升级版),中科院二区(基础版),IF = 5.349)
[9]Yuan, L., Guo, J., & Wang, Q. (2020). Automatic classification of common building materials from 3D terrestrial laser scan data. Automation in Construction, 110, 103017. https://doi.org/10.1016/j.autcon.2019.103017 (JCR一区, 中科院一区Top(升级版),中科院二区Top(基础版),IF = 10.517)
[10]Liu, P., Chi, H. L., Li, X., & Guo, J. (2021). Effects of dataset characteristics on the performance of fatigue detection for crane operators using hybrid deep neural networks. Automation in Construction, 132, 103901. https://doi.org/10.1016/j.autcon.2021.103901 (JCR一区, 中科院一区Top(升级版),中科院二区Top(基础版),IF = 10.517)
[11]Tang, X., Wang, X., Guo, J., Wang, Q., & Zhang, J. (2022). Benefits of Terrestrial Laser Scanning for Construction Quality Assessment: A Time and Cost Analysis. Journal of Management in Engineering (ASCE). 38(2), 05022001. https://doi.org/10.1061/(ASCE)ME.1943-5479.0001012 (JCR一区, 中科院二区(升级版),中科院三区(基础版),IF = 6.415)
[12]Li, J., Wang, Q., Ma, J., & Guo, J. (2022). Multi-defect segmentation from façade images using balanced copy-paste method. Computer‐Aided Civil and Infrastructure Engineering. 37(11), 1434-1449. https://doi.org/10.1111/mice.12808 (JCR一区, 中科院一区Top(升级版),中科院一区Top(基础版),IF =10.066)
[13]Qiu, Q., Wang, M., Guo, J., Liu, Z., & Wang, Q. (2022). An adaptive down-sampling method of laser scan data for scan-to-BIM. Automation in Construction, 135, 104135. https://doi.org/10.1016/j.autcon.2022.104135 (JCR一区, 中科院一区Top(升级版),中科院二区Top(基础版),IF = 10.517)
[14]Cui, Z., Wang, Q., Guo, J., & Lu, N. (2022). Few-shot classification of façade defects based on extensible classifier and contrastive learning. Automation in Construction, 141, 104381. https://doi.org/10.1016/j.autcon.2022.104381 (JCR一区, 中科院一区Top(升级版),中科院二区Top(基础版),IF = 10.517)
[15]Liu, P., Qi, H., Liu, J., Feng, L., Li, D., & Guo, J. (2023). Automated clash resolution for reinforcement steel design in precast concrete wall panels via generative adversarial network and reinforcement learning. Advanced Engineering Informatics, 58, 102131.
[16]Xiang, C., Gan, V. J., Guo, J., & Deng, L. (2023). Semi-supervised learning framework for crack segmentation based on contrastive learning and cross pseudo supervision. Measurement, 217, 113091.
[16]Xiang, C., Guo, J., Cao, R., & Deng, L. (2023). A crack-segmentation algorithm fusing transformers and convolutional neural networks for complex detection scenarios. Automation in Construction, 152, 104894.
[17]Deng, L., Zhang, A., Guo, J., & Liu, Y. (2023). An Integrated Method for Road Crack Segmentation and Surface Feature Quantification under Complex Backgrounds. Remote Sensing, 15(6), 1530.
[18]Chu, H., Long, L., Guo, J., Yuan, H., & Deng, L. (2023). Implicit function‐based continuous representation for meticulous segmentation of cracks from high‐resolution images. Computer‐Aided Civil and Infrastructure Engineering.
奖励与荣誉
2022 国家优秀自费留学生奖学金,中国国家留学基金委
2022 博士后国际交流计划引进项目(第一批),合作导师:邓露
2020 第十五届“春晖杯”中国留学人员创新创业大赛 优胜奖(最高奖项)
2020 第十二届“中国青少年科技创新奖”新加坡地区唯一候选人
2018 重庆大学优秀毕业生干部
(欢迎土木工程、工程管理、机械工程、计算机工程等相关专业,数学、计算机、外语基础好,对智能建造与运维及科研创新感兴趣的本科生或研究生加入团队。)